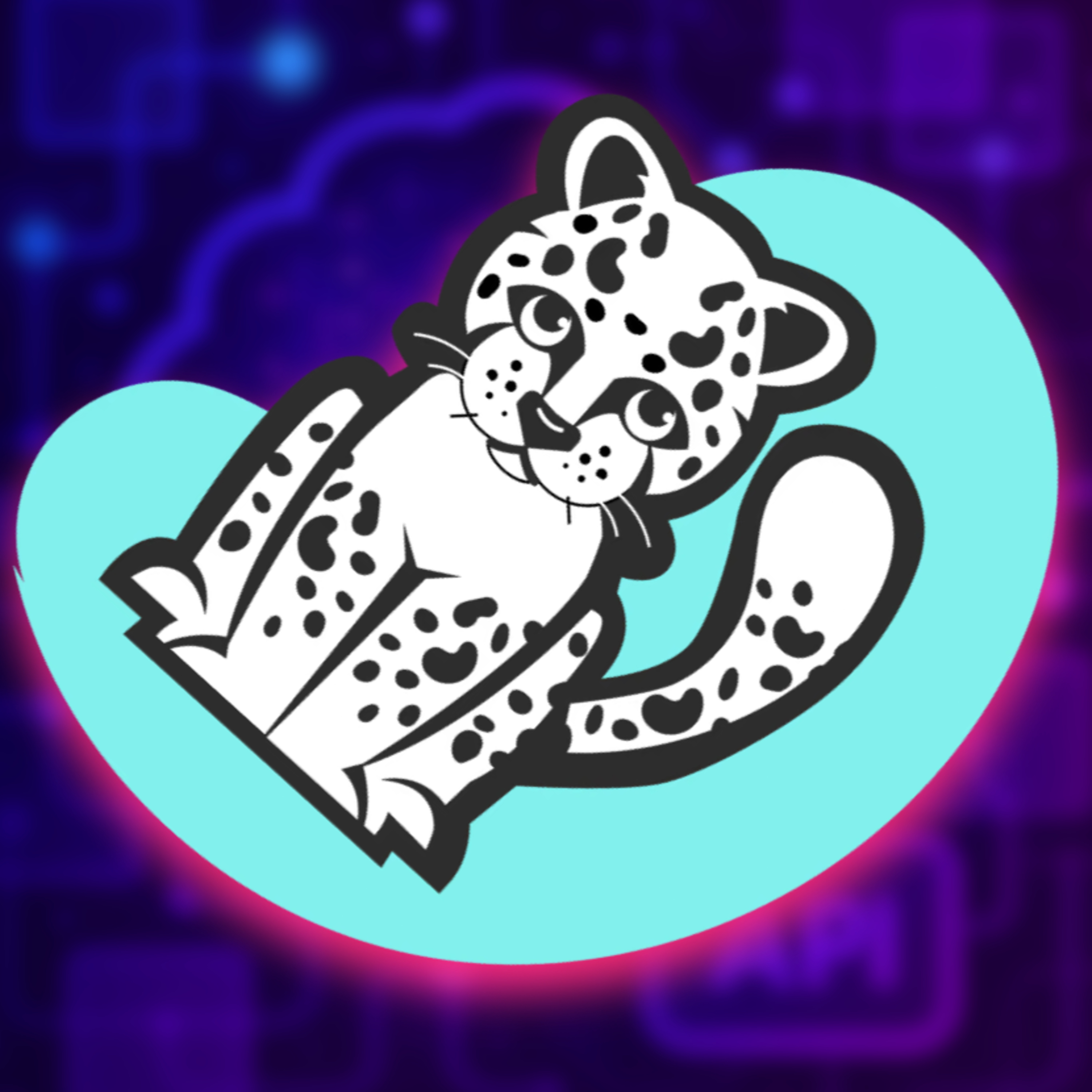
In Episode 99 of Great Things with Great Tech, Anthony speaks with Deepti Srivastava, founder of Snow Leopard AI and former founding PM of Google Spanner. Deepti explores how enterprise AI is often built on stale, siloed data and introduces a bold solution: real-time, federated access to live business data—no ETL, no pipelines, just direct answers. Snow Leopard AI enables structured data retrieval across SQL and APIs with AI-powered query generation, placing AI directly in the critical decision path.
We all talk about #AI, but what good is it if your models are powered by stale, outdated data?In Episode 99 of Great Things with Great Tech, Deepti Srivastava, founder and CEO of Snow Leopard, and former founding PM of Google Spanner, calls out the broken state of enterprise AI. With decades of experience in distributed systems and data infrastructure, Deepti unveils how Snow Leopard is redefining how AI applications are built, by tapping into live, real-time data from SQL and APIs without the need for ETL or pipelines.Instead of relying on static snapshots or disconnected data lakes, Snow Leopard’s #agentic platform queries native sources like PostgreSQL, Snowflake, and Salesforce on-demand, empowering AI to live directly in the critical decision path.In This Episode, We Cover:
- Deepti’s journey from building Spanner at Google to founding Snow Leopard AI.
- Why most enterprise AI fails due to reliance on stale data and outdated pipelines.
- How Snow Leopard federates live data across SQL and APIs with zero ETL.
- The limitations of vector databases in structured, real-time business use cases.
- Why putting AI in the critical path of business decisions unlocks real value.
Snow Leopard is a U.S.-based technology company founded in 2023 by and is Headquartered in San Francisco, CaliforniaSnow Leopard specializes in building a platform that enables the development of production-ready AI applications by leveraging live business data. The company's approach focuses on real-time data retrieval directly from sources like SQL databases and APIs, eliminating the need for traditional ETL processes and data pipelines. This innovation allows for more accurate and timely AI-driven business decision.
PODCAST LINKS Great Things with Great Tech Podcast: https://gtwgt.comGTwGT Playlist on YouTube: https://www.youtube.com/@GTwGTPodcastListen on Spotify: https://open.spotify.com/show/5Y1Fgl4DgGpFd5Z4dHulVXListen on Apple Podcasts: https://podcasts.apple.com/us/podcast/great-things-with-great-tech-podcast/id1519439787
EPISODE LINKS Snow Leopard Web: https://www.snowleopard.ai/Deepti Srivastava on LinkedIn:https://www.linkedin.com/in/thedeepti/Snow Leopard on LinkedIn: https://www.linkedin.com/company/snow-leopard-ai/
GTwGT LINKS Support the Channel: https://ko-fi.com/gtwgtBe on #GTwGT: Contact via Twitter/X @GTwGTPodcast or visit https://www.gtwgt.comSubscribe to YouTube: https://www.youtube.com/@GTwGTPodcast?sub_confirmation=1Great Things with Great Tech Podcast Website: https://gtwgt.com
SOCIAL LINKS Follow GTwGT on Social Media:Twitter/X: https://twitter.com/GTwGTPodcastInstagram: https://www.instagram.com/GTwGTPodcastTikTok: https://www.tiktok.com/@GTwGTPodcast
🎵 Music by BenSound: https://www.bensound.com
☑️ Support the Channel: https://ko-fi.com/gtwgt
(00:00) Most enterprise AI is built on stale data, and that's a problem. While companies brag about LLMs and chat bots, their critical decisions are still powered by outdated snapshots and clunky pipelines. In this episode of Great Things is Great Tech, we meet Deputy Shastava, who also gave us Google Spanner at Google Cloud Platform, and now is founder of Snow Leopard AI. (00:24) She's calling out the broken state of enterprise AI and showing us a new path. Building real time AI apps using fres h live data from SQL and APIs. No ETL, no delays, just answers. This is episode 99 of Great Things with Great Tech with Snow Leopard. Welcome to the show. This is amazing to have you on and to talk about Snow Leopard. Love the name by the way. (00:58) It's kind of amazing. We'll get we'll get into that a little bit later on in terms of the vision and to the name of the company. But before we get into what Snow Leopard does, um really interested in your background because you've it's quite fascin ating doing the research on you where you've come from um and what you've actually been part of in your professional career already. (01:15) It's quite amazing and I'm sure a lot of people who are out there have learned of have understood Google Spanner and they understand that that's a really big time platform in Google Cloud and you had a hand in doing that. But before we get to that, give us a little bit of background about yourself, where you came from and you know, effectively how you came to be in technology. (01:36) Yeah. Um, thank you so much Anthony. It's so great to be here. Um, and wow, that's quite the intro. So um yeah, I've been in technology in specifically in building data and production data systems and infrastructure for about two decades now. I feel old but um I say that as well now two 20 years. It's like I'm one of those guys now that says I've been in this gig like 20 25 years. (02:02) Yeah, I feel you. Yeah. But uh so I feel and in that time like you say I feel v ery fortunate because I've been part of not one but two platform shifts which is incredible. Um you know both the sort of cloud shift and the and the data analytics shift. Um and it's been amazing to to watch where software and applications and the whole sort of tech world has come in those past sort of two decades. (02:34) Um, and to to talk about sort of how I got here and who I am, like so, um, I've lived in sort of 10 different cities, give or take, on three continents. Um, at this point I f eel very much like a citizen of the world. Um, and I've learned so much about both people, about how technology impacts people, etc. in that time. Um, and so, so I went, like, you know, I grew up in India. (03:02) I went to grad school in Carnegie Melon. Uh so so in the US in Pittsburgh and um I started studied computer science in England. So I definitely sort of like hopped around um and at grad school I was researching how you you make this interconnected world of the internet um work. Like ba sically my my thesis topic was distributed fault tolerant systems which is now what we call the cloud. (03:30) So I feel very fortunate that you know me and my cohort were sort of part of building that sort of bringing cloud to life which is very exciting and like you know for everyone sort of infrastructure seems boring and for me there's two things one I'm really excited about infrastructure because it's the enabler like a that's where all the sort of ambiguous problems are and sort of the lik e well how do you really make this work like those kinds the questions come up and so that's always been exciting to I know like we're (04:04) talking about data infrastructure. It's like, oh, so boring. But to me, it's like, oh, that's like that's where the crown jewels of every every business, every sort of everything is. Data is really important. And so, I've really been excited about that. And the second thing has which has driven my career. And I didn't have the words for this earlier, but but I really care about how technology impacts people. (04:27) Um, and and sort of sub like unconsciously or whatever. I've my my career has gone from like an engineer like a systems engineer at Oracle like in the Oracle kernel you know building sort of distributed locking et etc to like as you say spanner to Google where I switched to being essentially a product person to like um you know what I'm doing now which is running a company but it's it's sort of that trajectory has been about like wel l how is what what I'm working on what I'm building impacting humans right yeah that's sort of been what's driving me. Yeah, I love (05:00) that as well. And I love that you talk about infrastructure. I think a lot of the talk around modern day computing is around cloud and software and AI and what that is, but there's obviously something that backs that and that's infrastructure, right? So, I love that you are focusing there on you mentioned it. (05:19) I love the the analogy around the crown j ewels of of every uh enterprise data creation has to live somewhere, has to be created somewhere. You have to interact with something. You got to run some tooling, no matter where that is, whether it's onrem or the cloud. It is infrastructure that runs that. So, I love that you focus in on that. (05:37) And then your other part about helping people, you know, become better or businesses become better and doing more with technology. Quite clear in that sense. It's interesting. So Oracle you so th at's some really deep stuff that you were doing at Oracle right that sounds pretty deep you know over over my head but then from the you know how did you get from Oracle to go to Google and then what was the sort of genesis of working around Google Spanner because as I'm as I've sort of mentioned before Google Spanner is kind of popular and in fact I (06:06) know that from a uh veh perspective when I'm out talking to customers I'm a product manager at the moment as well there we go where's the w here's our gaps oh Google Google Spanner. Can you do Google Spanner? We get that quite often, right? So, it's obviously found its way into enterprise in that NoSQL big data sort of scenario. (06:26) So, the impact is ongoing from your perspective. So, well done there. But how did you get there? Like what was that kind of connection? Yeah. Wow. Um I think what was motivating me I think going back to your point about Oracle, right? Like I I went from grad school to working on the only sort of you know that level of deep distributed system that I could find at the time like that I knew of like which was you know Oracle rack is even today actually I was looking at DB engines is is the largest installed database in the world even today right so in terms of (07:03) like impacts like that's pretty pretty big right and like it's not like trivial stuff it's like you know your airlines run on it and like your banks run on it and like your healthcare runs on it right so it's It's pretty interesti ng and ubiquitous technology. However you feel about the company and and whatever, I think the technology itself is pretty cool. (07:23) And to to be able to be in the in the lock like if you're in in a database, everything is a lock. And so when you're managing distributed locking, it's like you actually get to see almost everything. All the bugs sort of stopped there, etc. So I had a really interesting bottoms up view of how enterprises worked, right? like so I how does at the time like how do es you know this airline industry do ticketing and and when it fails like what are the sort of knock-on effects of that so um that was really interesting but but as an engineer like I was going (07:54) pretty deep like vertically down but I didn't really know apart from when bugs came in what what that technology was being used for why why is it interesting why is it in the critical path etc right and so so I think that's what drove me to Google where I was looking for well how can I know the en d to end cradle to grave of a particular product right and spanner at the time I don't think the paper was published I think it was it was still in the works but um but or maybe it was I don't remember this is like late 2012 early (08:25) 2013 um so so that was the like they were building something from the ground up that was supposed to scale almost infinitely but was a trans so a transactional database transaction scale. Yeah. Which is I think the key difference here, right? Like we were not t rying to say we're going to be semi-transactional or we're going to be eventually consistent, right? Like we were supposed to be like in fact Spanner has linearizability and serializability for for the nerds and geeks about like consistency out there. And that was (08:58) really interesting to me. I'm like, okay, why are we doing this? What what are we doing? So, so I went to Google and my my sort of nuclear project was to launch Spanner internally and then get all the sort of products that that are Google products that everybody's familiar with and more onto Spanner, right? And the reason was because um we were using my SQL for ads database was which at the time was obviously the the key to Google's sort of whole business. (09:27) we were having to like Google was having to shard it and quietest it every six to 18 months, right? And and each shard adding was taking between six and 18 months. So it was like this really um complicated system and and nothing out there in the world was ab ve those billion users like really impacts the end user? Like I had a because I was now looking at the full end to- end product. (10:58) It was a really exciting and amazing learning opportunity around how to build technology products. Yeah. And I think that's really interesting. I had the I was talking about my previous episode which went live this week as this episode is recording and it was like the product that everyone uses but no one really knows I use it was real time analytics. (11:19) I you know um first of all thank you that is very kind. I really to be honest I felt special being part of that team and being the product person for that product. It just it today also like it was such a special group of people trying to solve such a interesting problem like you know having that confluence of interesting problem interesting people interesting time when the world was actually scaling up right like Google was ahead in the scale game but like the world was also scaling up and so it ight it's which is different from eventual consistency which is like maybe you'll see if I deposit 50 bucks maybe Maybe you see 50 bucks, maybe you (13:50) see a 100 bucks because there was 50 bucks already in the Yep. Yep. Yep. bank account or maybe you see zero, right? Because we don't know if I withdrew 50 or if you withdrew 50, etc. So, so that's really important from like a user perspective like you need to know what the money was in your account. (14:08) There is no like let's see what hap shadowing a little bit where we are today in terms of data being super critical and where a lot of these uh generative AI platforms and AI in general and everything really everything it's all sucking data out from effectively these particular platforms right like they're so significant in today's world and I guess this is where we transition to snow leopard a little bit and talk about that (15:30) um in 2023 established and founded this company but what what there's a bit of a gap there to sort snow leopard so that's a very interesting question um I went from Google to um you know be the head of product for this data visualization company called observable um so it's like a little bit you know it's in the sort of data application stack rather than like in the infrastructure layer which was again a really interesting (17:04) um place to be cuz a I was like oh well I have I've built infrastructure but I want to see how how it's used right like how how do we service it so going up one lev feels like Google's finally kind of not so much caught up, but kind of taking the rightful place in terms of where they should have been. (18:14) And everyone was kind of bagging them off and saying, "Oh, why why Google struggling here?" It's like, well, just wait a little bit. And I think we're starting to see that, especially from what I've seen this week in terms of the recording date. But anyway, go on. No, I I like I think we Google's always built really awesome technology and to to see the w CTO's at sort of midsize companies that I sort of had came across. So I just was like hey how are you using this? And it turns out that you know everybody was trying to figure out how to go from PC to production. Like PC's were cool but production was like not happening. (19:43) And so I wasn't really like sort of looking for like for a problem or a solution. I was just like, how are people using it in my true like, you know, just how does technology impact people? So, how is this technology i e you really using it in business decision making? And in my experience, if you put a technology here, I know we're a podcast so you can't see me justiculate, but Oh, that's it's visual as well. (20:59) But if you put it on the side and not in the middle critical path, then you're not going to derive the trillion dollar value of AI that everybody talks about, right? And so that's sort of what engendered the thought around, you know, building the data platform. I mean, I'm gonna build build a pla his technology and make it um work in that critical line like you talked about? And I guess the the point that you you I know that I've kind of come across in the last few months is that getting the data out of these data lakes, these platforms, these structured systems is actually challenging. not so much challenging in (22:32) that you can't get it out because everyone can can write a query and pull the data out, but it's about how efficient and how smart you get that out to impact a real-time o like from the Indian region. (23:40) Um which is a hearkening back to my roots. And then they actually make amazing they're one of the best mammal moms and I'm a mother etc. So this was all like stuff that to me is personal. Um, and I wanted the platform to be something that exists, is strong, is agile, is fastm moving. They're very fast moving that can support this fastmoving world of AI and be strong and versatile for the AI developers, but not be in your face all the time, right? Like it's o yourself personally and then at the end to come and say that you kind of validated this through Kung Fu Panda and that makes it even more amazing. (25:19) So no that that's cool. So let's talk about very quickly the platform you know how it's different from others where's the innovation here. I mean what I'm reading into it is that you know it it basically eliminates uh the need for predefined data pipelines ETLs that sort of stuff which from my understanding again I'm not in that data science that are facts of life in my opinion when it comes to data and I think you with your day job probably relate to them, right? One is data silos are a fact of life, right? There is always a data silo out there. No matter how beautiful, big, amazing your data lake and and data warehousing solution is, there's always a data source that you have not thought of, that you have not etlled in and is out there. (27:11) Number one, right? So then this this thought process around and this is before AI like al path when you're talking about recommendation systems right like recommendation systems may fail but you can still serve the answer so so one of the things is like for this technology LLMs and AI and all that to exist and really have an impact on businesses and users at the end of it like you have to put it in the in the critical path that's number two (28:31) and then number three is like what you said right let's say you've built the most beautiful system maintaining it, managing it. It's t e and it's like going to be um the most performant it can be because we're quering it in the native language. (30:00) Okay. So what if I were to give you an example? Can I give an example? Yeah. Of course. Yeah. I'm I'm painting a picture in my head and I'm kind of working out an interpret the a central interpreter kind of man in the middle situation. But yeah, give me give let's let's hear it from you as an example. (30:17) I think that will help. Yeah. So I think normally how this is done let' on't think it works for structured data. The second part of this, the vector store, is that it's a fuzzy matching system. So you've taken 40, 50, 60 years of of database research, right? Where you give very precise answers and you get yes or no answers, right, from a SQL system or an API and you've now turned it into a fuzzy matching system, which is like you've lost all the data context, all the schema, like all that stuff is lost, (31:50) right? Like it's no longer an accurate system and then I want. So is that is that really helping me in terms of the outcome that I want to your point and I think you've articulated again that problem that I' I've always thought about vector searches um quite well. (33:01) Anyway, continue. Thank you. Yeah. No, I I think that again as a as a person who's spent their entire life building, you know, these deterministic systems to go from there to like, you know, if I'm looking for Anthony and and let's say this last name is SPI Mhm. and and not your f data retrieval which means we figure out which which place to go in real time. (34:34) Then we build the Postgress query and the Salesforce query or the snowflake query in real time in the native language. So PGSQL, sle and snowflake SQL. Then we go run those queries on those systems. Then we when the data is sort of returned, we retrieve it back and give it back to you. Okay? Right? So um so instead of consolidating all the data we are doing a federation approach right so we can get the freshe nd obviously in the middle of that that's the that's the brains that's the smartest of of Snow Leopard is the fact that you've got sort of an agentic potential system. You're probably leveraging elements of AI to create those um native queries as they're required, which I think is really cool as well. (36:20) Um I I toyed a little bit actually with um doing a asking the LLM to create a Postgress query on the fly depending on what the question was or the prompt was and then it natively it got giv it's on steroids but this agentic system understands business logic at a gen general scale right so each developer doesn't have to build their own agents to do that and then we're using essentially deterministic system programming to build those query on the fly. Good stuff. (37:50) We got about two and a bit minutes left. I just had a quick thought on that. So, is it customizable per client that you're going to bring on or is it Yeah. You So, you'll work with the with the customer to make it's upport, etc. That's awesome. (39:00) Yeah. Um, got a minute left. Um, usually we talk about futures, but I feel like we've already talked about where this is going and what it is and what's in the what's in the future for the general industry and where the problem is and where we are actually tracking the solution. So, in a couple of seconds, tell us a little bit more about, you know, where where is Snow Leopard going from here? Any other information you want to give? Um, because you are early, uned for more episodes where we continue to highlight companies and technology shaping our world. Don't forget to follow us on social media, gtwgtodcast and visit gtwgt.